AI is the Cure for Broken Health Care Systems
AI's much needed role in healthcare towards optimizing hospital logistics, reducing physician burnout, enhancing disease detection, and improving patient care.
Good health is a privilege, not a guarantee.
That’s why healthcare is unique as an industry that we will all interact with throughout our lives, whether we like it or not.
Beyond the sisyphean task of day-to-day patient care, healthcare is a creaking sector of ancient IT systems, poor tech integration, mountains of siloed data and manual processes crying out for automation.
It’s also one of the most exciting for innovative AI applications, as technology is finally maturing to a point where it can make a meaningful impact for patients, hospitals and beyond.
In this post, I want to highlight some use cases I think are overdue for their time in the AI sunshine. Thanks also to my colleague Bertram Weiss, VP Health at Merantix Momentum, for providing his input.
Hospital Logistics and Bed Management
Inefficiencies in bed allocation, medication supply chains, and staff scheduling all lead to wasted resources and delayed patient care.
Bed availability is one of many operational pain points for hospitals. But where administrators see a chaotic mess of complex data and poorly integrated systems, AI can streamline and provide actionable insights, as a 2019 Harvard Business Review found.
A John Hopkins Hospital implemented an AI-powered control center that improved bed assignment efficiency by 30%, reduced the need to keep surgery patients in recovery rooms longer than necessary by 80%, and cut the wait time for beds for incoming emergency room patients by 20%.
AI is also being trialled in transforming predictive inventory control, particularly for medications and critical medical supplies. AI-powered time series forecasting models, including ARIMA (AutoRegressive Integrated Moving Average) and LSTMs (Long Short-Term Memory networks), anticipate fluctuations in medication demand, helping hospitals prevent stockouts and avoid costly overstocking.
A study published in Acta Logistica evaluated the performance of ARIMA and LSTM models in forecasting the demand for five commonly supplied medicines in Rwanda's public health system. The LSTM model demonstrated superior accuracy, achieving a 95.2% performance rate in demand prediction, indicating its potential to significantly reduce stockouts and overstocking.
If you’ve ever tried to get a doctor to give you a firm answer on whether they can attend a future event, you will know how astoundingly chaotic hospital staffing methods are. Days off have to be booked months in advance and understaffing means those precious days off for overworked medical staff can simply disappear into thin air.
Fortunately, AI-driven reinforcement learning algorithms are improving hospital staffing models, dynamically adjusting workforce deployment based on expected patient inflows, peak hours, and emergency events. Bioengineering Basel’s 2024 report demonstrated that AI applications could predict patient admissions and discharges, allowing for dynamic staffing adjustments. This proactive approach helps in maintaining optimal nurse-to-patient ratios, thereby improving patient care and reducing staff burnout. The report also suggested that this data could help improve patient experience by giving accurate information about appointment times, wait periods and treatment schedules.
AI-driven scheduling is also cutting costs and improving efficiency in the operating room, according to a 2024 report from the Fort Lauderdale Eye Institute.
A machine learning algorithm designed to optimize operating room booking times led to a 21% reduction in nursing overtime, resulting in significant cost savings.
Over a three-year period, the reduction in overtime hours translated to $469,000 in savings, highlighting AI’s potential to alleviate staff burnout and improve operational efficiency.
These improvements aren’t just reducing costs—they are fundamentally reshaping hospital management by ensuring that every resource, from beds to staff to equipment, is utilized at peak efficiency, directly improving patient outcomes and hospital profitability.
AI-Powered Medical Scribes
Doctors spend a third of their working hours filling in paperwork, a Hett Insights report found. It doesn’t stop when they clock off either, with some spending nearly 5 hours of their personal time per week wrestling with documentation. This is a staggeringly poor use of time that contributes towards burnout and something that can easily be taken over by AI scribes.
AI scribes leverage automatic speech recognition (ASR), natural language processing (NLP), and large language models (LLMs) to extract key information from conversations in real time. AI-generated notes follow standardized formats such as SOAP (Subjective, Objective, Assessment, Plan) and BIRP (Behavior, Intervention, Response, Plan), ensuring consistency and accuracy, according to an NEJM Catalyst study.
A pilot study on AI scribes in dermatology found that they significantly reduced documentation time, enabling doctors to focus more on patient interactions instead of administrative tasks. The American Medical Association (AMA) reported that AI scribes could save doctors one hour on average, drastically improving efficiency and reducing burnout among healthcare professionals.
AI scribes aren’t just a helpful notetaker for doctors making their rounds, they’re also a valuable source of health record and insurance data that can be integrated into future multimodal AI.
Multimodal AI will allow for data from text, such as doctor’s notes and EHRs, to be examined along with images and time series data like X-rays, MRIs and monitoring data from wearables and hospital equipment. AI will assist doctors by quickly synthesizing complex patient histories and identifying correlations that would take a human much longer to process.
Scribes have huge potential to cut back on paperwork and increase the time doctors share with their patients. Any opportunity to automate bureaucracy faced by clinical staff should be taken up by innovators with the dual goal of helping to increase patient rate and generate a valuable data pool from the associated claims data that can help with personalized patient interventions, disease forecasting and working towards a 360 degree multimodal approach to patient care.
AI for Epidemic Detection and Pandemic Preparedness
The global spread of COVID-19 laid bare the grim reality of pandemic preparedness. Thankfully, the worst of the coronavirus is in the rearview mirror, but experts warn that we are far from prepared for the next global outbreak.
The bad news is that we don’t know when the next one will strike. The good news is that AI might be our best chance to minimise the impact when the time comes.
A February 2025 study from the University of Oxford highlights the transformative role of AI in predicting, detecting, and containing pandemics before they escalate. The study found that AI-powered epidemiological modeling, genomic tracking, and real-time surveillance are essential tools in strengthening global pandemic preparedness:
• Improving current models of disease spread, aiming to make modelling more robust, accurate and realistic.
• Pinpointing areas of high-transmission potential, helping ensure limited healthcare resources can be allocated in the most efficient possible way.
• Improving genetic data in disease surveillance, ultimately accelerating vaccine development and the identification of new variants. Real-time surveillance using genomics such as PCR means wastewater can now be monitored as one of the earliest sources for detecting pathogens, something that was successfully implemented during the pandemic.
• Helping determine the properties of new pathogens, predict their traits and identify whether cross species jumps are likely.
• Predicting which new variants of already-circulating pathogens – such as SARS-CoV-2 and influenza viruses – might arise, and which treatments and vaccines are best in reducing their impact.
• Possible AI-aided integration of population-level data with data from individual-level sources – including wearable technologies such as heart rate and step counts – to better detect and monitor outbreaks.
• AI can create a new interface between the highly technical science and healthcare professionals with limited training, improving capacity in settings that need these tools the most. This can bridge between staff that went into the sector for the ‘human interaction’ and help speed up the relatively slow adoption of technology in healthcare.
AI is also proving essential in vaccine development, where it accelerates genomic analysis and drug repurposing, cutting traditional vaccine research timelines by up to 50%. Pharmaceutical firms such as Moderna and Pfizer are actively integrating AI into their R&D processes, allowing for faster identification of high-risk mutations and more precise vaccine targeting.
Epidemiologists are quick to remind us that the next pandemic won’t politely wait around the corner until we’re ready: it’s only a matter of time before the next infectious disease takes hold. The time for AI adoption must be between pandemics, not during one.
In my book, that’s a pretty urgent call to action. For ambitious builders and healthcare experts looking to create tools that will help manage the next pandemic, yesterday was the best time to start, today is the next best opportunity.
AI-Powered Wearables and Health Monitoring
AI-enabled wearable devices are revolutionizing real-time health monitoring, providing continuous, non-invasive insights into patient well-being. These wearables offer early detection of health conditions, personalized interventions, and enhanced patient care. Recent research highlights their growing role in cardiovascular health, human activity recognition, and intensive care monitoring.
A 2023 study showed that wearable ECG devices demonstrate high efficiency in processing single-lead ECGs. The research shows that the devices can deliver near real-time cardiac assessments in 33 to 35.7 seconds, ensuring faster diagnosis and early intervention. These ECGs improve arrhythmia detection and reduce reliance on manual interpretation, helping healthcare professionals manage cardiac patients more effectively.
A 2025 study published in npj Digital Medicine found that a deep learning model trained on a dataset of over 150,000 individuals found associations with 1,600 diseases, outperforming traditional ECGs:
“When compared to standard ECG intervals, the autoencoder-based latent space models reveal a substantially greater number of associations, suggesting that the rich representations afforded by the architectural complexity of the deep learning model provide more information about disease status than routinely ascertained ECG intervals.”
The paper also notes that consumer-based ECG devices could enable large-scale, remote disease detection.
This powerful predictive is backed up by another study from the American Heart Foundation which reported that devices in the ‘Multisensor Monitoring in Congestive Heart Failure (MUSIC) study’ developed and validated a personalized algorithm to predict acute decompensation in 543 patients with heart failure. The wearable device predicted impending hospitalization with 76% to 88% sensitivity at a median of 6.5 days before hospital admission. That’s a huge window for preventative action.
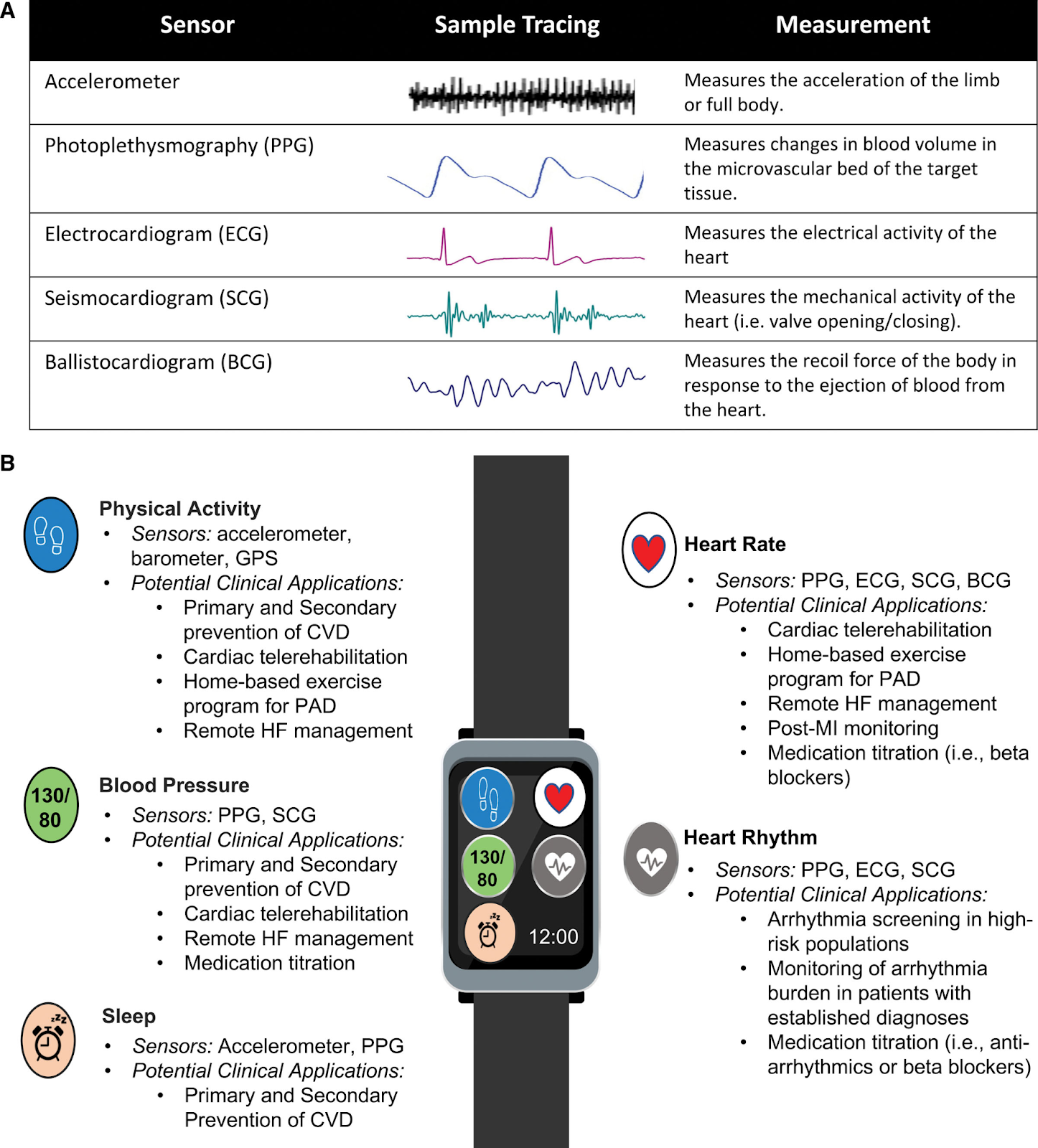
These wearables are also a sign of another trend: building systems outside of traditional healthcare infrastructure.
Traditional infrastructure, such as hospitals and surgeries, often use outdated information systems such as EHR/EMR and in some cases wouldn’t be able to integrate live data from wearable devices. That’s why some innovators are trying to reinvent healthcare end-to-end: to allow the AI to work to its fullest potential and rethink the experience entirely. It’s also another reason why it is easier to set up this independent infrastructure in prevention.
Just look at Spotify co-founder Daniel Ek’s new AI-powered full-body scan and preventative healthcare platform, Neko Health. The firm’s clinics use AI and non-invasive sensor technology to generate millions of data points across cardiovascular, metabolic, and dermatological health indicators. Patients receive comprehensive AI-assisted insights into potential early-stage health risks such as cancer and cardiovascular disease.
They say it’s the next step towards preventative health, although I think there are some fairly large structural and incentive challenges in the health industry before doctors start prescribing jogging and earning from healthy patients rather than sick ones. That said, a pilot test is underway in Kinzig Tal doing just that.
Either way, wearables have enormous potential to improve the doctor’s ability to diagnose and treat patient’s illnesses, in some cases allowing for preventative action. On top of this, new AI tools could connect siloed databases and insurance code to improve tracking in wearables and immediately send alarming data to doctors. We will also see new kinds of sensors that are able to monitor different variables. This unprecedented availability of patient data should be mined to bring real-time nowcasting into healthcare and make trend-spotting, diagnosis and treatment as dynamic as they can be.
An industry more than ripe for AI
Healthcare isn’t just another industry—it’s a system we all rely on that remains crippled by inefficiencies and missed opportunities.
AI presents a rare opportunity to rebuild critical processes from the ground up, not just optimize broken ones. For founders and innovators, this isn’t about incremental improvements; it’s about reshaping how care is delivered, predicted, and personalized around the world.
The first stages of preventative health are finally within reach, with AI wearables catching cardiac events before they happen and predictive models identifying disease risks early. Hospitals are no longer bound by guesswork, as machine learning streamlines logistics, staffing, and resource allocation. Meanwhile, AI-driven automation is freeing doctors from paperwork, returning them to patient care where they belong.
The challenge now is execution. Legacy infrastructure, regulatory barriers, low IT budgets and entrenched incentives won’t make AI adoption seamless - but they do create massive openings for those ready to build smarter, faster, and more patient-centric systems.
Healthcare won’t fix itself. The innovators who recognize this moment as an inflection point will define the next decade of medicine. I’m looking forward to seeing— and helping drive - this transformation.
Thank you Rasmus, a very good overview!.
I would add, even if is countertuitive ...
AI will help us "humanize" healthcare...
If done well. ;)